Miguel Liu-Schiaffini
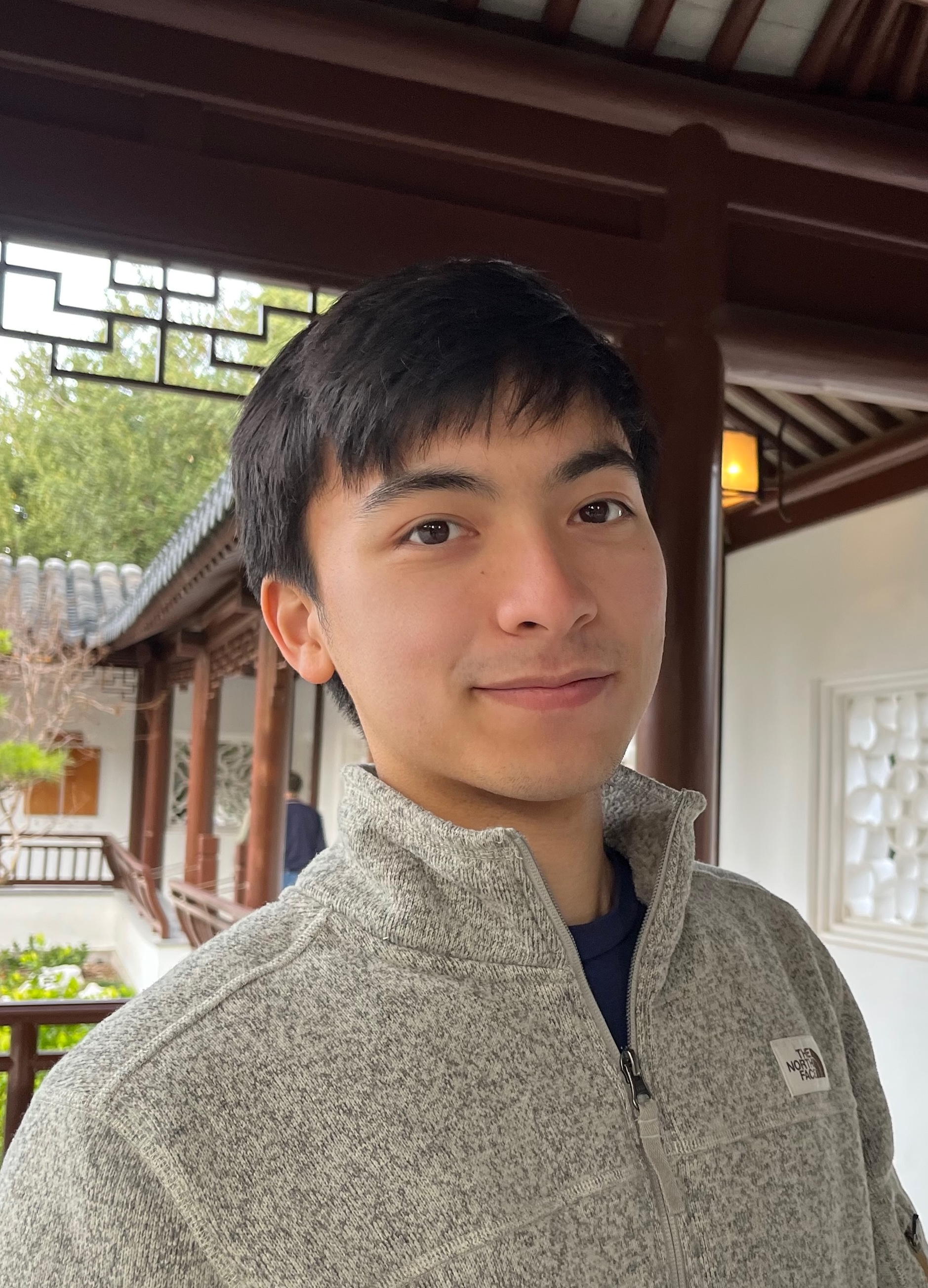
I am a fourth-year undergraduate at Caltech, studying computer science. Currently, I am a research intern in the Learning and Perception group at NVIDIA Research and a member of Anima Anandkumar’s AI + Science lab at Caltech. In the past, I was also a research intern at the University of Texas Institute for Geophysics.
My current research lies in the theory and applications of neural operators and operator learning, particularly in their application to solving partial differential equations (PDEs). For instance, I have worked on developing neural operators for forecasting in chaotic, non-stationary, and stochastic time-dependent systems. I am very passionate about the scientific applications of machine learning and have worked on using machine learning to forecast climate tipping points, estimating glacial ice thickness, and characterizing features of the Martian terrain.
During my undergrad, I have been very grateful for support from the Mellon Mays Undergraduate Fellowship. I was also a recipient of the 2024 Barry Goldwater scholarship.
News
Oct 23, 2024 | Our new paper, “Unifying Subsampling Pattern Variations for Compressed Sensing MRI with Neural Operators” is on arXiv! |
---|---|
Sep 20, 2024 | I gave an invited talk at the 2024 Human-Centered AI Conference on tipping point forecasting on function spaces. Many thanks to Prof. Fabien Scalzo for the invitation! |
Jul 21, 2024 | I presented our recent papers “Neural Operators with Localized Integral and Differential Kernels” and “Towards Enforcing Hard Physics Constraints in Operator Learning Frameworks” at ICML 2024 in Vienna! |
Jun 17, 2024 | I am starting my internship in the Learning and Perception research group at NVIDIA research! |
May 15, 2024 | I have been named a recipient of the 2024 Henry Ford II Scholar Award! I am very grateful for the support of all my mentors and from the computer science department. |
Selected publications
- Neural Operators with Localized Integral and Differential Kernels41st International Conference on Machine Learning, 2024
- Neural Operators for Accelerating Scientific Simulations and DesignNature Reviews Physics, 2024
- Ice Thickness From Deep Learning and Conditional Random Fields: Application to Ice-Penetrating Radar Data With Radiometric ValidationIEEE Transactions on Geoscience and Remote Sensing, 2022
- Tipping Point Forecasting in Non-Stationary Dynamics on Function SpacesarXiv preprint arXiv:2308.08794, 2023
- Towards Enforcing Hard Physics Constraints in Operator Learning FrameworksICML 2024 AI for Science Workshop, 2024
- Unifying Subsampling Pattern Variations for Compressed Sensing MRI with Neural OperatorsNeurIPS 2024 Workshop on Machine Learning and Compression, 2024